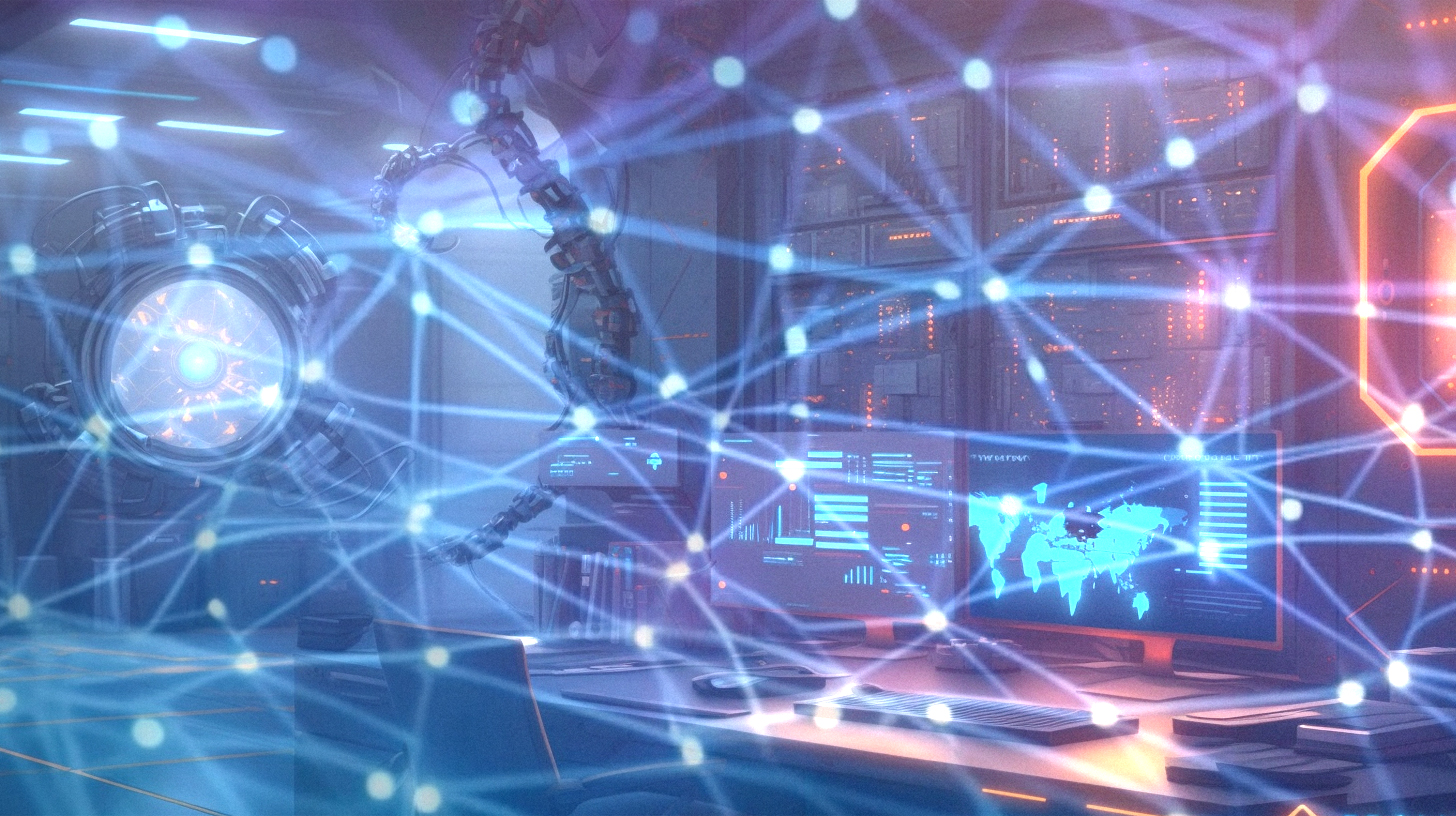
In this blog article we are going to take a small deep dive into the topic of mathematical graph-based models and look at the benefits they can provide for an efficient cyber risk analysis management. Readers will gain an understanding on why graph-based models can be a valuable tool for OT security and how adding context to enrich graph-based models can gather the desired insights.
What are graph-based models – literally and metaphorically speaking?
Graph-based models visualize relationships among objects or substances via edges and nodes, which are the main components of any graph model. Nodes are points whereas edges are lines that highlight the connections between said nodes. The multiple connections among various nodes displayed by many edges create an image of interconnected points – commonly referred to as a graph. To put it briefly, graph-based models generally consist of various nodes, which are connected to each other via edges (lines), thus creating the familiar graph structure as depicted below.
Within a graph-based model every node is a visual symbol for an object whereas edges are a simplified representation of established relationships among these objects (nodes). When thinking of a production (OT) environment for instance, the nodes can be the OT assets (e.g. machines, hardware, software components etc.), while the edges represent the various connections between these OT assets.
A plausible edge joining two assets in an OT environment could be the digital communication process between two machines (nodes) via IO Link (Safety) for example. Other conceivable edges depicted in a graph-based model can symbolize inner or outer connections to networks among several OT assets.
The purpose of graph-based models:
In general, graph-based models operate as a representation of variables and their interrelationships. They can serve as a simple visualization of various kinds of networks in an organization such as the IT infrastructure or an OT setting.
The point of having a graph-based visual is to showcase via nodes and edges how data is arranged in a real-life setting. Simply put, graph-based models can help us envision and understand highly complex surroundings. Furthermore, the utilized data behind the graph-based models are rooted in contextual variables (from a multitude of data sources), that can evolve over time, thus making a situational analysis as well as a cyber risk simulation tenable. Exemplary contextual data (variables) for a sufficient OT security analysis are CVEs, CVSS, HSE factors, amount of assets, number of segments or segment size. Therefore, graph-based models can be additionally utilized to break down complex (would be) scenarios (e.g. the inheritance potential among various OT assets or segments in case of a cyber-attack) since the conditional arrangement between variables allows these graphs to function as a probabilistic model.
The benefits of graph-based models when analyzing complex systems
As systems are becoming increasingly elaborate, the risk potential grows as well. OT assets for example are becoming more digital. Machines within the OT environment are progressively communicating with each other all in an effort to automize the production site and thus improve process efficiency with the aim of having the best output. Given the growing complexity of digital systems, potential avenues of harm, when it comes to cyber-attacks are:
- Inheritance risk
- Distance to known risks
- HSE factors (HSE = Health Safety Environment)
- Inner and outer connections
- Segment Size
- Amount of (digital) assets
In the context of IT/OT security, graph-based models are particularly useful when analyzing intricate systems and the interdependences as well as relations found within said system. Consequently, a graph-based model analysis tool can support with uncovering hidden risks, that have the potential to fortify and therefore, increase damage.
Understanding one’s OT environment – What more can be done with graph-based models?
Without the usage of graph-based models one may prioritize the wrong issue within the IT/OT environment. In addition, it may be more difficult to pinpoint exactly what the matter is and how it can be addressed due to the lack of illuminating multidimensional view when overseeing IT/OT infrastructures in an organization.
Implementing graph-based models in one’s cyber risk assessment will establish an orderly structure because these insights will aid in finding and evaluating the root cause that contributes to manifesting certain risks. Furthermore, graph-based models enables you to identifying how certain risks relate to one another and why. As every OT environment is uniquely designed and there is (metaphorically speaking) “no one size fits all”, a cyber risk analysis methodology that can be customized to one’s specific environment is even more important.
In summary, graph-based models can help one understand their own production environment, by clarifying connections across various nodes (assets) via edges. They reflect real life structures and relationships in multiplex realms such as the OT environment. By implementing a risk analysis technique rooted in graph-based mathematical models, one gets the metaphorical bigger picture while also being able to pinpoint exactly the respective variable(s) causing the issue.
Author: Fabienne Okafor